TensorFlow Layers for NLP: Part 1
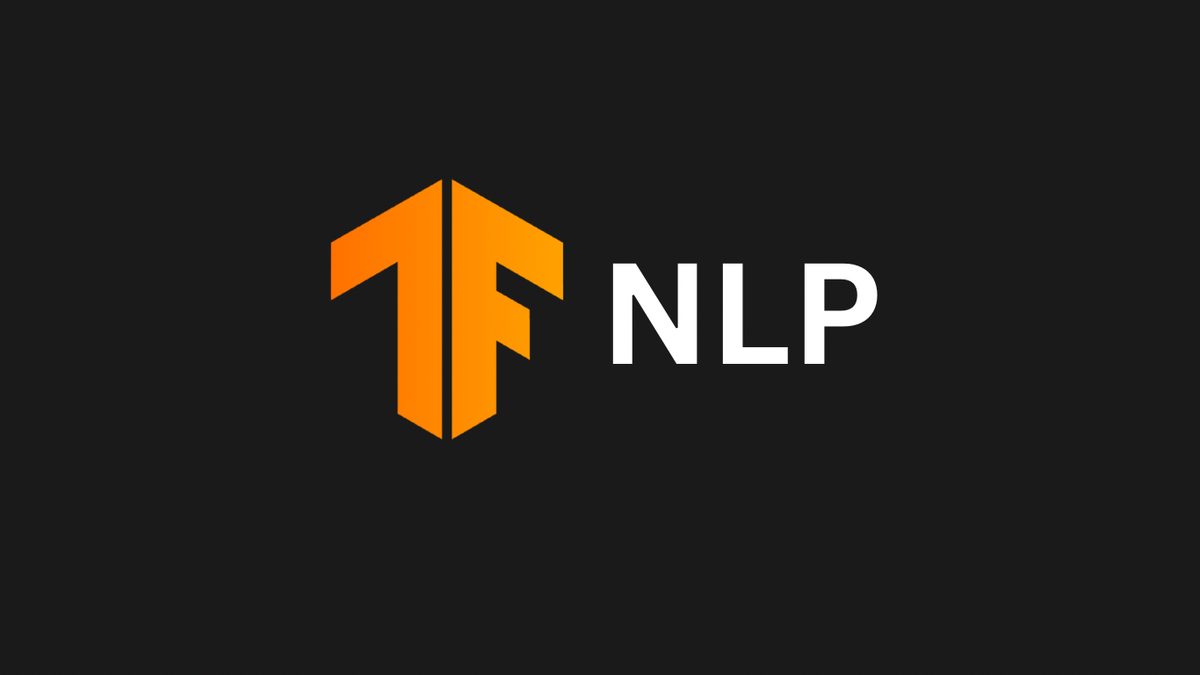
Introduction
From biology to code. While we are still too far away from real resemblance of human intelligence in AI, the roots have already been planted. ML/DL models consisting of layers is a simplification of how we see human brain or to be more precise - its neurons, connections, and impulses. Humans took thousands of years of evolution to develop complex understanding of a language that consists of a combination of smaller tasks such as sentiment, parts of language, sequences, relationships, categorizations, entity recognition, and many many more. This is what model layers, and their combinations, intend to do - tackle particular tasks.
In this article, you will learn about:
- Understanding the crucial role of layers in NLP
- Impact of layer choices on dimensionality
- Key layers like Embedding, Flatten, Global Pooling, Dense, Recurrent (LSTM), and TimeDistributed
- Practical examples using TensorFlow for hands-on learning that uncover what it looks like under the hood
- Tips for layer selection
Don't forget to check the Jupyter Notebook that breaks down what can be found inside each layer, and what insight we might want to look for in it.
Why Layers Matter
The Foundations of Intelligence
In a neural network, layers are akin to the neurons in the human brain. Just as neurons process and transmit information, layers in a neural network perform specific operations on the data they receive. In Natural Language Processing (NLP), these layers are crucial for unraveling the intricacies of human language, spanning semantics to context.
Versatility and Adaptability
NLP tasks are diverse, ranging from text classification and sentiment analysis to machine translation and question-answering systems.
Different layers offer different capabilities, making them more suited for specific tasks. For instance, Recurrent Neural Network (RNN) layers are excellent for sequence-based tasks like machine translation, while Attention layers have proven invaluable in tasks requiring context-awareness.
Efficiency and Performance
Choosing the right layers can significantly impact the efficiency and performance of an NLP model. For example, Embedding layers in text representation tasks can drastically reduce the dimensionality of the input data, making the model faster and more memory-efficient.
The Key to Generalization
Layers like Dropout and Batch Normalization play a crucial role in preventing overfitting, making the model generalize better to unseen data. This is particularly important in NLP, where the model needs to understand and adapt to various linguistic nuances.
The Power of Composition
The true power of layers comes from how they can be combined. Just like words form sentences and sentences form paragraphs, layers can be stacked and interconnected to create complex NLP models capable of understanding language at a deeper level.
Layers Breakdown
Embedding Layer
In NLP, words or phrases are often represented as discrete tokens, which are not directly suitable for mathematical operations. The Embedding layer serves as a translator, converting these tokens into continuous vectors. These vectors capture the semantic meaning of the words, making them amenable to machine learning algorithms.
Embeddings layer is essentially a lookup table, where each row in the weights matrix corresponds to a word (it's number in the vocabulary to be more precise) in the input data. You'll find a specific example in the notebook.
If you are new to word embeddings, I recommend you check out my article just on that: Transforming Words into Meaningful Vectors.
Wait, when we use Word2Vec for creating embeddings, do we need to use Embedding layer in Tensorflow then?
The short answer is yes. First of all, by using Tensorflow layers we are able to use its native functionalities for seamless training process. And we can transfer Word2Vec embeddings straight to Embedding layer weight matrix since both are look up tables for words in a vocabulary. The interesting part is that we can mark the Embedding layer as either trainable or non-trainable. Marking it as trainable is useful when we want to use pre-trained embeddings from a Word2Vec model to fine-tune them with task specific input text in our new model.
import tensorflow as tf
# Assuming word2vec_model is a gensim Word2Vec model
embedding_matrix = word2vec_model.wv.vectors
embedding_layer = tf.keras.layers.Embedding(
input_dim=embedding_matrix.shape[0],
output_dim=embedding_matrix.shape[1],
weights=[embedding_matrix],
trainable=True # Set to False if you don't want to fine-tune the embeddings
)
Why do we even have both? Are they interchangeable?
Word2Vec was specifically designed to learn word associations. For example, the model can learn that "bark" is associated with "dog". Tensorflow's Embedding layer can be tuned to any specific task. For example, by training a model in Tensorflow specifically for sentiment analysis, the Embedding layer will develop associations for this particular task.
However, if a training dataset is small, using a pre-trained Word2Vec model for the start might be a better idea. Then, we can fine-tune it with our smaller dataset by configuring the layer as trainable.
Flatten & Global Pooling Layers
Overview
The purpose of these layers is to simplify the high-dimensional output from the previous layers and flatten them into a 1D array. It is often used as an intermediate step after layers that process high-dimensional data such as Convolutional or Embedding layers, and before Dense layers.
Note: in the images below, for now focus on what effect Flatten layer has on the dimensionality of data. If you don't understand Dense layer yet, don't worry. We'll cover it a bit later, it's not important in the example below.
This image illustrates what happens to the multi-dimensional data that is fed into a Dense layer without flattening it out first:
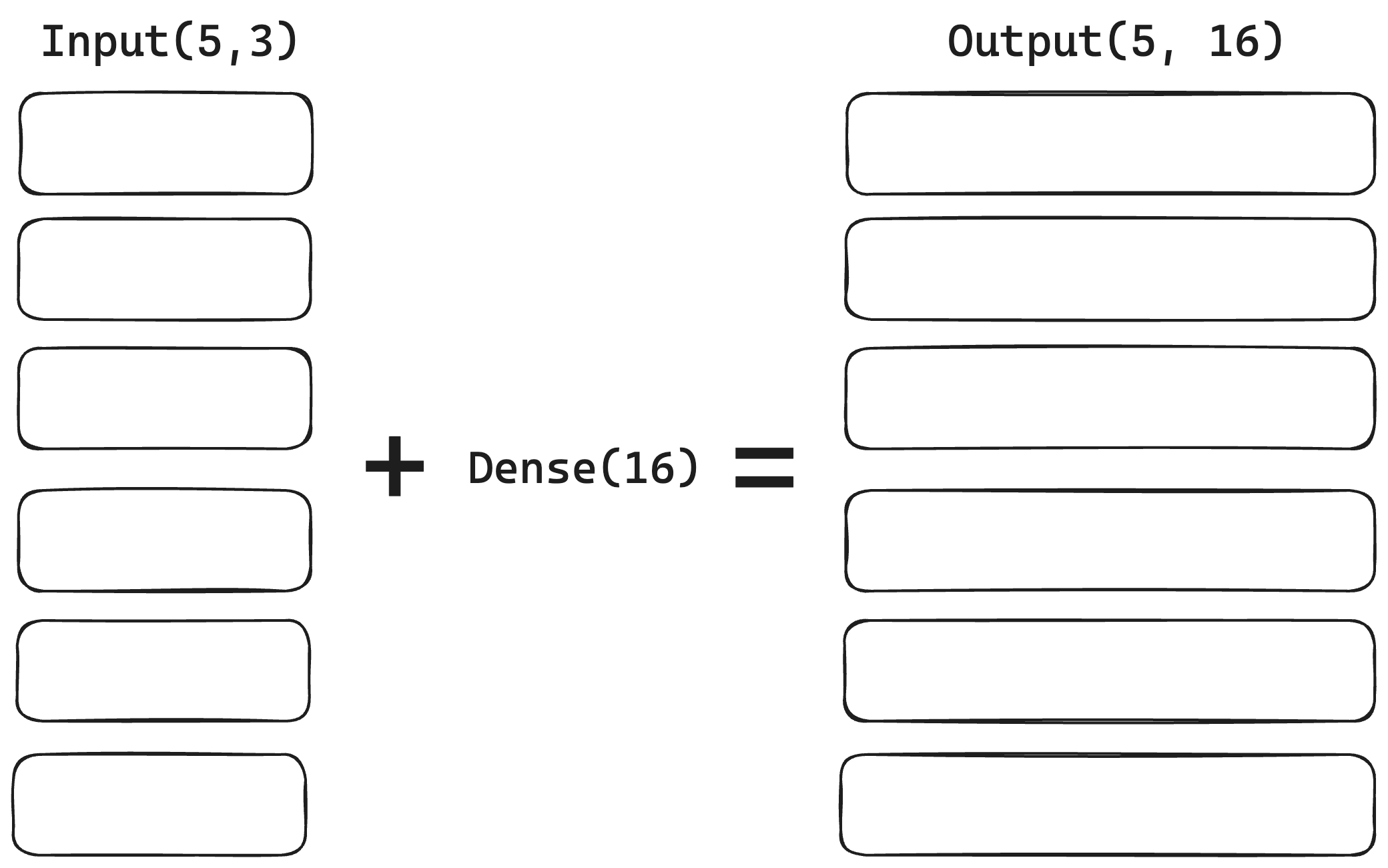
Now, how would the output look like if we applied Flatten layer before passing it to Dense layer:
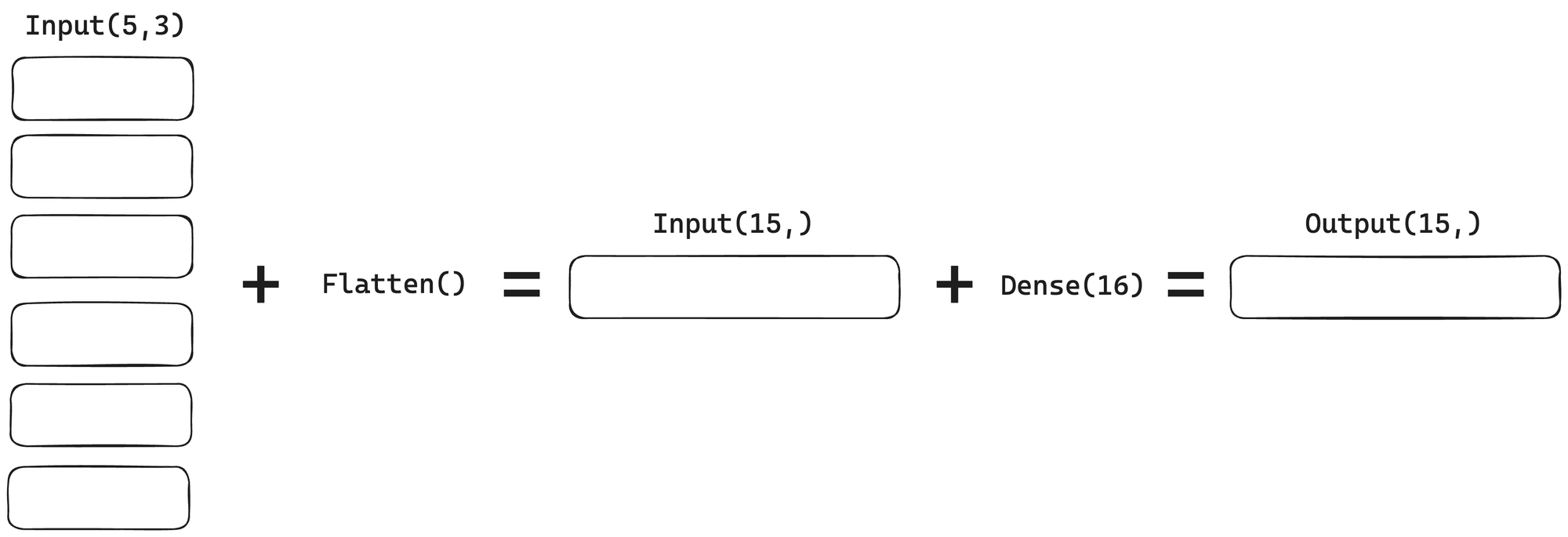
Flatten reduces the number of dimensions, but keeps all the features that are now just lined up in one array instead. It only reshapes the multidimensional data.
There are some alternatives: GlobalAveragePooling1D and GlobalMaxPooling1D.
The GlobalAveragePooling1D layer calculates the mean over the time dimension (0 axis, meaning vertically).
The GlobalMaxPooling1D layer selects the maximum value over the time dimension.
Examples
Here is the comparison of all 3 layers. Let's say we have two reviews and their respective embeddings:
"Great movie"
- "Great" → [0.9, 0.8]
- "movie" → [0.7, 0.6]
"Bad acting"
- "Bad" → [0.1, 0.2]
- "acting" → [0.3, 0.4]
And it would look like this after passing it to the Embedding layer:
[
[[0.9, 0.8], [0.7, 0.6]], # Review 1: "Great movie"
[[0.1, 0.2], [0.3, 0.4]] # Review 2: "Bad acting"
]
Next, we process this data using one of the layers we just discussed:
Flatten
[
[0.9, 0.8, 0.7, 0.6], # Flattened Review 1 combined horizontally
[0.1, 0.2, 0.3, 0.4] # Flattened Review 2 combined horizontally
]
GlobalAveragePooling1D
[
[0.8, 0.7], # Average of Review 1 along 0 axis
[0.2, 0.3] # Average of Review 2 along 0 axis
]
GlobalMaxPooling1D
[
[0.9, 0.8], # Max values from Review 1 along 0 axis
[0.3, 0.4] # Max values from Review 2 along 0 axis
]
Risks Associated With These Layers
While flattening and pooling layers provide seamless transition from convolutional or recurrent layers to densely connected ones, it possess certain risks. By doing so we might jumble up useful information. In the realm of NLP this might disrupt the order within a sequence which might lead to the loss of information such as sentiment and relationship. This might result in inaccurate understanding of data by the model, which will result in poorer performance.
Dense Layer
Overview
Dense layers, also known as fully connected layers, are the most straightforward yet versatile layers in neural networks. In NLP, they often serve as the final layer for tasks like text classification or sentiment analysis, mapping high-level features extracted by previous layers to output labels.
A Dense layer connects each input node (or neuron) to each output node, with each connection containing a weight. It essentially learns patterns and relationships from the features passed to it from previous layers during a training process. The layer learns weights and uses an activation function to produce an output.
In NLP, a Dense layer often comes after feature extraction layers such as Embedding or Convolutional layers.
Example
Let's consider a real-life scenario where we are building a sentiment analysis model to classify movie reviews as either positive or negative.
Here are two short reviews and their respective embeddings:
"Amazing film"
- "Amazing" → [0.9, 0.8]
- "film" → [0.7, 0.6]
"Poor storyline"
- "Poor" → [0.1, 0.2]
- "storyline" → [0.3, 0.4]
After passing through an embedding through GlobalAveragePooling1D, we get:
[
[0.8, 0.7], # Average of Review 1
[0.2, 0.3] # Average of Review 2
]
Now, these vectors are passed to the Dense layer with a 'relu' activation function. This layer learns to recognize patterns and relationships in these features to help classify the sentiment of the reviews.
For instance, it might learn that higher values in the first dimension of the embedding is often associated with positive or negative sentiment, and use this information to weigh the inputs accordingly.
Recurrent Layers (LSTM, GRU)
Overview
Recurrent layers are designed to recognize patterns in sequences of data. They maintain a kind of 'memory' that captures information about what has been calculated so far.
- LSTM: It has a complex structure with three gates (input, forget, and output) that control the flow of information to be remembered or forgotten at each time step. This helps in capturing long-term dependencies in the data.
- GRU: A simplified version of LSTM, with two gates (reset and update), making it computationally more efficient but potentially less expressive in capturing complex patterns.
Imagine we are building a sentiment analysis model to classify sentences from movie reviews as positive or negative.
Here are two short reviews:
- "The movie had excellent visuals and a gripping storyline."
- "I found the plot quite dull and the acting mediocre."
In the first review, it might recognize the positive sentiment from the words "excellent" and "gripping", and similarly, identify the negative sentiment in the second review from words like "dull" and "mediocre".
So, why do we even need to use a recurrent layer to capture the relationship if the Embedding layer already contains the information about the relationship between words?
The Embedding layer captures the relationship between words to transform text data into static numerical representation so it makes sense and not just random, while recurrent layers maintain a dynamic 'memory' of the context as they move through the sequence. This allows them to understand the changing context within a sentence.
For example,
- in the Embedding layer, the word "king" would be close to "queen" in the vector space because they both refer to royalty.
- and in the LSTM layer, in the sentence "The movie was not good", it could learn to understand the negation introduced by "not" which manipulates the sentiment of "good".
Recurrent layer units are sometimes also called features as they are learned values representing different features of input data. For instance, in the sentiment analysis use case, a model could learn that people tend to set the sentiment in their reviews in the beginning of the sentence. This is how an LSTM layer could "see" features within a sentence:
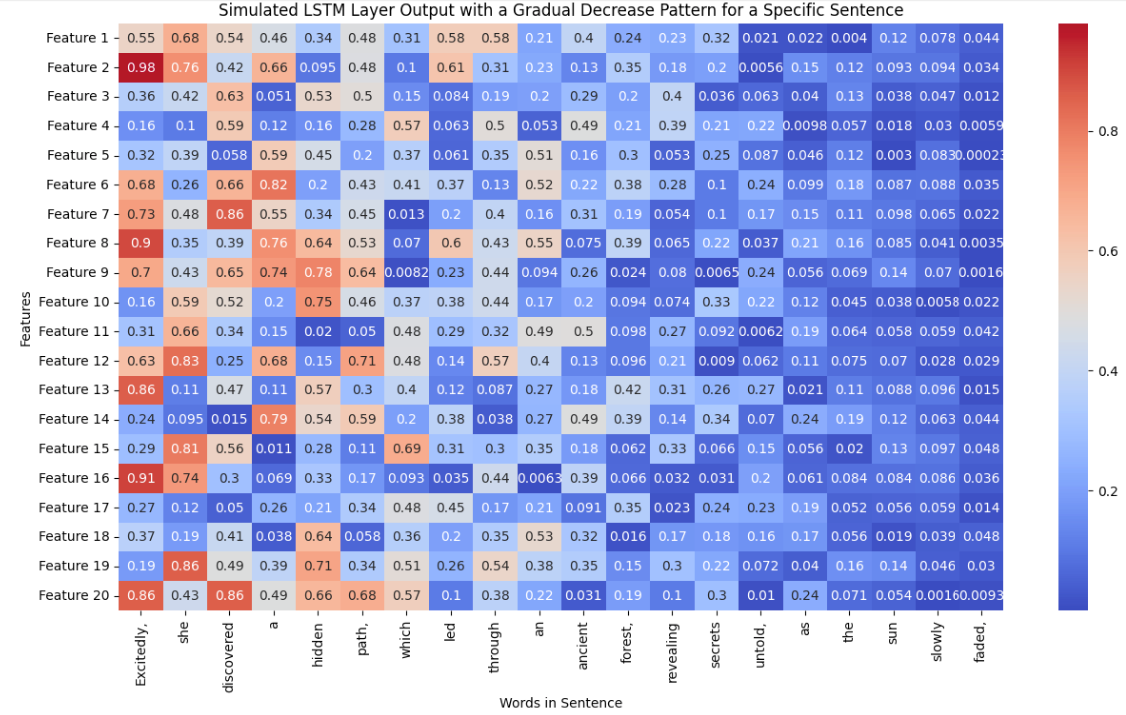
As you can see, the higher values tend to be mostly in the beginning of a sentence. What hat be an indication of a positive sentiment may vary, but for the same of this example we can assume the higher the value the more positive the sentiment is.
TimeDistributed Layer
Overview
The TimeDistributed layer is essentially a wrapper that you can apply to any layer, for instance Dense layer, allowing it to be applied to every time slice (or step) of a sequence independently. In the context of NLP, a "time slice" often refers to an individual word or token in a sentence.
What it Does:
- Preserves Temporal Structure: When you're working with sequential data like sentences, the order of the words matters. The TimeDistributed layer helps in maintaining this order by applying the same operation (like a Dense layer) to each word independently, but still considering it as a part of the sequence.
- Parallel Processing: Instead of processing the sequence word by word (or step by step), it allows the model to process all words (or steps) in parallel, which can make the training faster.
- Specific Predictions: In tasks like named entity recognition (NER) or part-of-speech tagging, we want to make a specific prediction for each word in a sentence. The TimeDistributed layer facilitates this by making independent predictions for each word.
Practical Examples
Dive into a hands-on demonstration of TensorFlow layers using the Tensorflow v2.13.0 library in my Python notebook. It shows how to use above mentioned layers. In certain cases, just not to repeat itself, the output of a particular layer is simulated in order to show you how it can be used and interpreted when actually trained on real data. Explore the code and examples in detail:
Tensorflow Layers for NLP: Jupyter Notebook Part 1
A Few Last Words
Understand the Problem Space
Before diving into layer selection, it's crucial to have a clear understanding of the problem you're trying to solve. Is it a classification problem like sentiment analysis, or a sequence-to-sequence task like machine translation? What kind if data patterns we might want to emphasize more or to be pivotal for our solution, and what is less relevant? The nature of the problem will guide your layer choices.
Start Simple
When in doubt, start with simpler layers like Dense and Embedding layers. These are versatile and can serve as a good starting point for many NLP tasks. Once you have a baseline model, you can experiment with adding more specialized layers and tune their parameters.
Sequence Matters
For tasks involving sequences like time-series analysis or text summarization, Recurrent layers like LSTM and GRU are often the go-to choices. They are designed to capture temporal dependencies, which are crucial in these tasks.
Context is King
If your task requires keeping and understanding the word order (e.g., question-answering, machine translation), consider using Attention layers. I will create another article just on this topic. They excel at capturing contextual relationships between words, even those far apart in the sequence.
Regularize to Generalize
If your model is overfitting, consider adding Dropout or Batch Normalization layers. These layers help in regularizing the model, making it more robust and better at working with new data.
Experiment and Iterate
Don't be afraid to experiment with different types of layers and combinations. Machine learning can get quite complex. Use validation metrics to gauge the effectiveness of different layer choices and don't hesitate to go back to the basics.
Leverage Pre-trained Models
For many NLP tasks, you can leverage pre-trained models that already include a well-thought-out architecture of layers. Fine-tuning these models for your specific task can save time and yield excellent results.
Conclusion
There are plenty of layers that can be used together for tackling specific language-related tasks. The key to success lies within precision of choice of layers for your task, clean and rich input data of course, and thoughtful experiments leading to continuous improvement. And just to give you an idea of how many layers Tensorflow offers us, visit its website for the complete list. But don't worry, I'll explain more layers in future articles. Remember, there is a lot of information out there, and going step by step will lead to great outcome.