Word2Vec Explained: Transforming Words into Meaningful Vectors
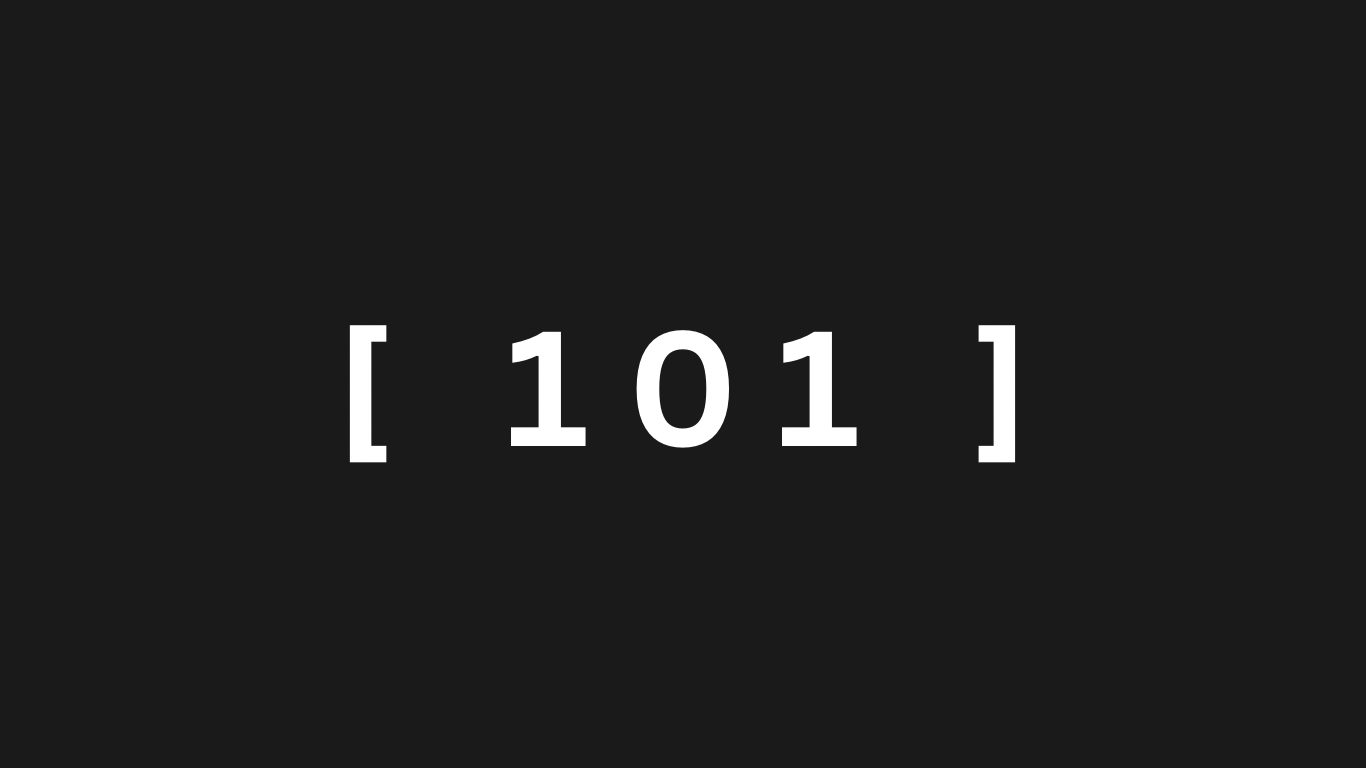
Introduction
Word2Vec, pioneered by Tomas Mikolov and his team at Google, has revolutionized the way we represent words in machines. Their groundbreaking work, presented in two 2013 papers (Efficient Estimation of Word Representations in Vector Space and Distributed Representations of Words and Phrases and their Compositionality), introduced a method that could capture the essence of words in dense vectors.
In this article, you will learn about:
- What Word2Vec is
- Advantages over Bag of Words & TF-IDF
- Word Embeddings
- Vector Arithmetics & Other Vector Operations
- Two Architectures: Continuous Bag of Words & Skip-Gram
- Word2Vec in Python with Gensim library
- Visualization of Word Vectors (definitely check this one out!)
By the end, you'll grasp the WHY, WHAT, and HOW of Word2Vec, guiding you to decide how to apply Word2Vec to your problems.
What is Word2Vec?
At its core, Word2Vec is like a translator, converting human-readable text into a language machines understand better: vectors. In more technical terms, Word2Vec is a technique that uses a shallow neural network to capture word associations in a large corpus of text, creating what we call word embeddings.
Now, you might wonder, why not stick to traditional methods like Bag of Words (BOW) or Term Frequency - Inverse Document Frequency (TF-IDF)? Well, Word2Vec offers some compelling advantages:
- it captures semantic relationships between words
- with its dense vectors, it is less sparse and less computationally expensive than traditional methods
- it takes into account surrounding words, hence understands context
- it generates vectors for words not seen during training, hence can infer "meaning"
However, it is not a "cure-all" wonder technique and it falls short in working with homonyms - words with the same spelling but different meanings. Take 'fly', for instance. Is it an insect buzzing around or the action of soaring through the air? Word2Vec might get puzzled.
Word Embeddings
Word embeddings are a collection of word vectors that were produced by the Word2Vec neural network. A vector is a numerical representation such as [1, 2, 3]. Each element in it is a dimension.
Think of dimensions as features or attributes. Let's use Jack and Emma as an example. Jack is 26 and stands at 1.75 m, while Emma is 30 and is 1.6 m tall. If we were to represent their age and height as vectors, Jack would be [1.75, 26], and Emma would be [1.6, 30]. Here, 'height' and 'age' are dimensions that help us differentiate between the two.
Height | Age |
---|---|
1.75 | 26 |
1.6 | 30 |
By looking at these features, we can distinguish between Jack and Emma.
Now, let's consider Word2Vec, which deals with words instead of people. Word2Vec attempts to identify features or dimensions based on how one word relates to another in a text corpus. It creates N abstract dimensions that are initially assigned random numbers. This is because the model starts with no prior knowledge about the relationships between words.
D1 | D2 | ... | D(N-1) | D(N) |
---|---|---|---|---|
0.4 | 0.3 | ... | 0.7 | 0.4 |
1.3 | 0.7 | ... | 0.1 | 0.7 |
During the training process, these vectors are adjusted based on the context in which words appear. Words that appear in similar contexts will have similar vector representations. It's important to note that these dimensions are abstract and don't correspond to clear semantic or syntactic properties such as 'height' or 'age'. They are learned from the data and are used to capture a wide range of contextual relationships.
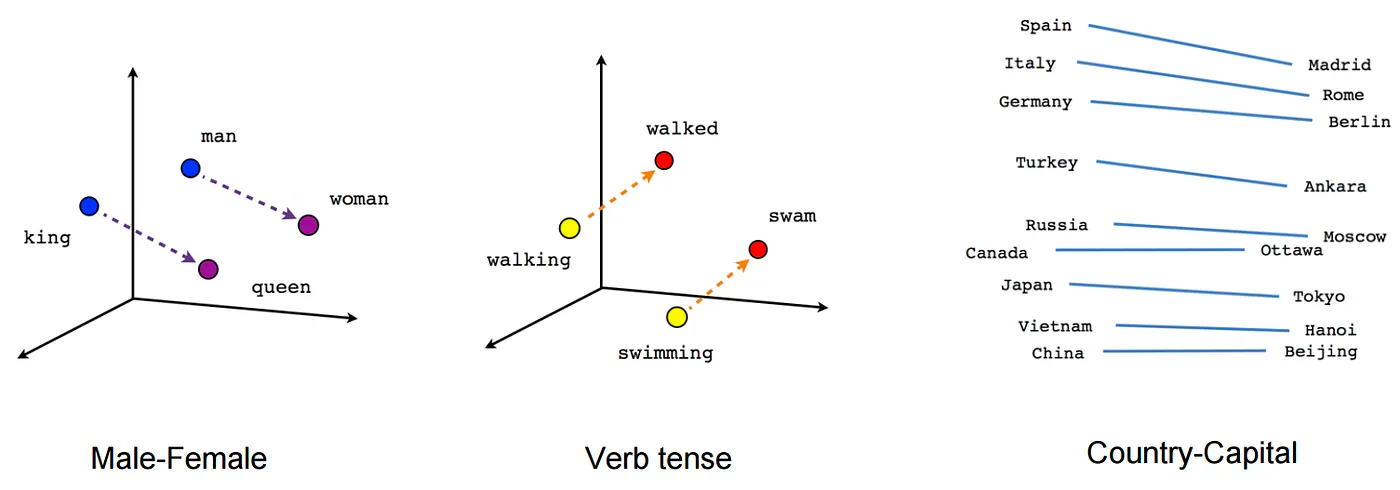
Word2Vec typically uses hundreds of dimensions to represent each word. This high-dimensionality allows the model to capture a wide range of contextual relationships.
Word2Vec Architectures
Word2Vec is a collection, or a family, of different models. Two of the most common model architectures are Continuous Bag of Words (CBOW) and Skip-Gram.
Note: Bag of Words (BOW) and Continuous Bag of Words (CBOW) are not related in the scope of this article. BOW is a feature extraction technique, whereas CBOW is a model architecture for learning word embeddings. BOW represents text, CBOW produces word embeddings. Two different things, two different purposes.
Continuous Bag of Words (CBOW)
Architecture Overview
CBOW is designed as a feed-forward neural network that takes into consideration surrounding words in order to predict the target word (also called the focus word).
The neural network consists of three main layers: the input layer, a hidden layer, and the output layer.
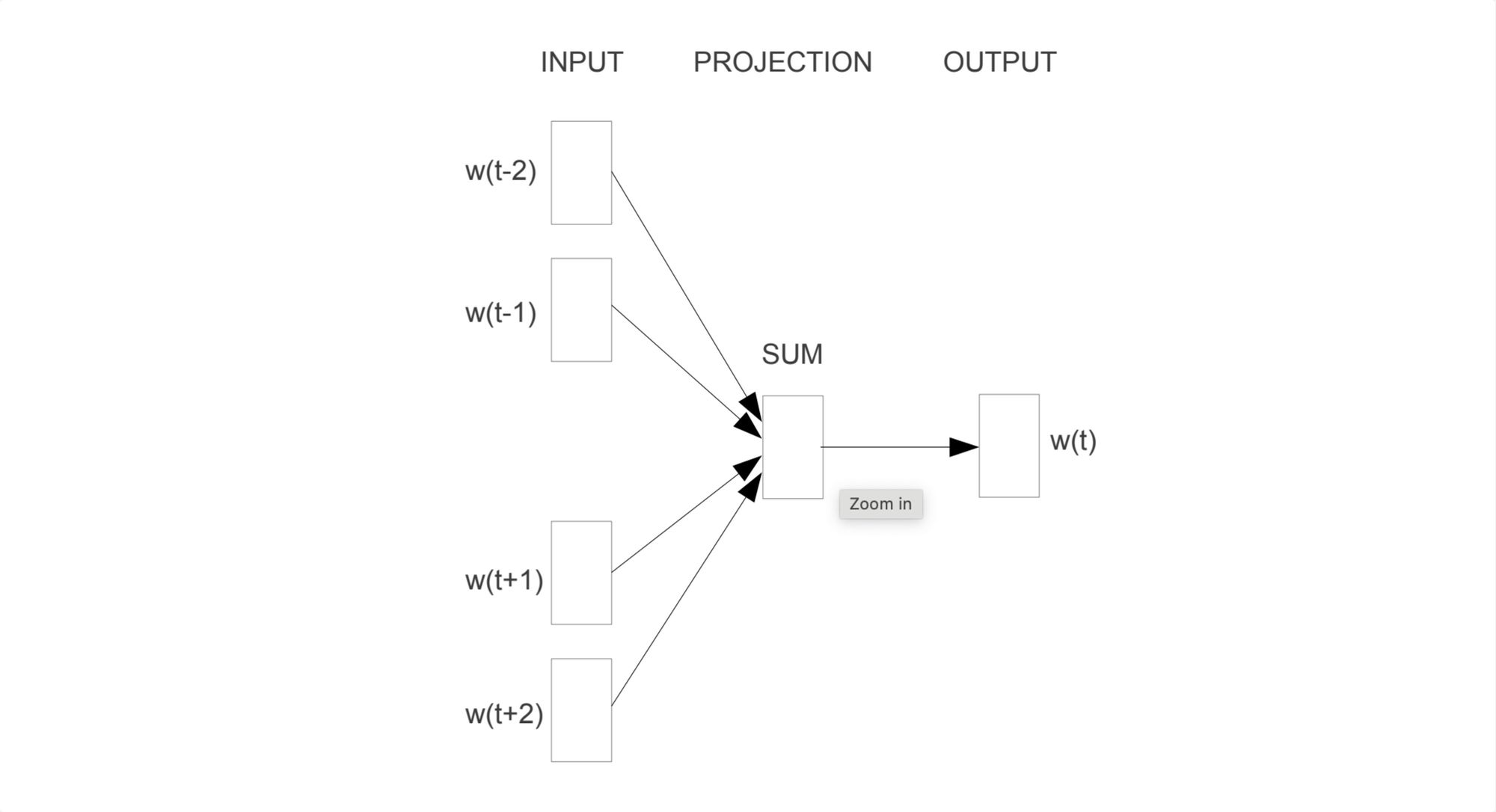
Input Layer
- Context Words: These are the words around the target word, which we aim to predict. They are encoded as one-hot vectors.
The input size is determined by:
- Vocabulary Size: This dictates the length of the one-hot encoded vectors. For instance, with a vocabulary of 10,000 words, each one-hot vector will have a length of 10,000.
- Sliding Window Size: This determines how many context words (and thus vectors) are fed into the network simultaneously. If the window size is set to 4, the model will intake four one-hot encoded vectors at once.
After being one-hot encoded, the context words are forwarded to the hidden layer.
Hidden Layer
- This layer aggregates the one-hot encoded vectors from the input layer, typically by summing or averaging, to produce a single consolidated representation.
- It usually comprises several hundred neurons, often around 300.
- The weights connecting the input to the hidden layer serve as the word embeddings for the context words. As the model trains, these weights adjust to capture the semantic relationships between words, and thus they effectively become dense representations (or embeddings) of words.
- Typically, the activation function here is linear or identity.
Output Layer
- This layer uses the softmax activation function, with a neuron count equal to the vocabulary size.
- It outputs probability distribution over the entire vocabulary, indicating the likelihood of each word being the target word.
Training Objective
The goal during training is to adjust the model weights to maximize the probability of the target word given its context.
Example
I want to predict what is a quick cat more likely to do outside the house: sit, run, sleep etc. The neural network would try to infer that based on given context: ("quick", "cat", "outside", "house"). Given the adjective "quick" and the context of being "outside" the house, it's logical to infer that the cat might "run".
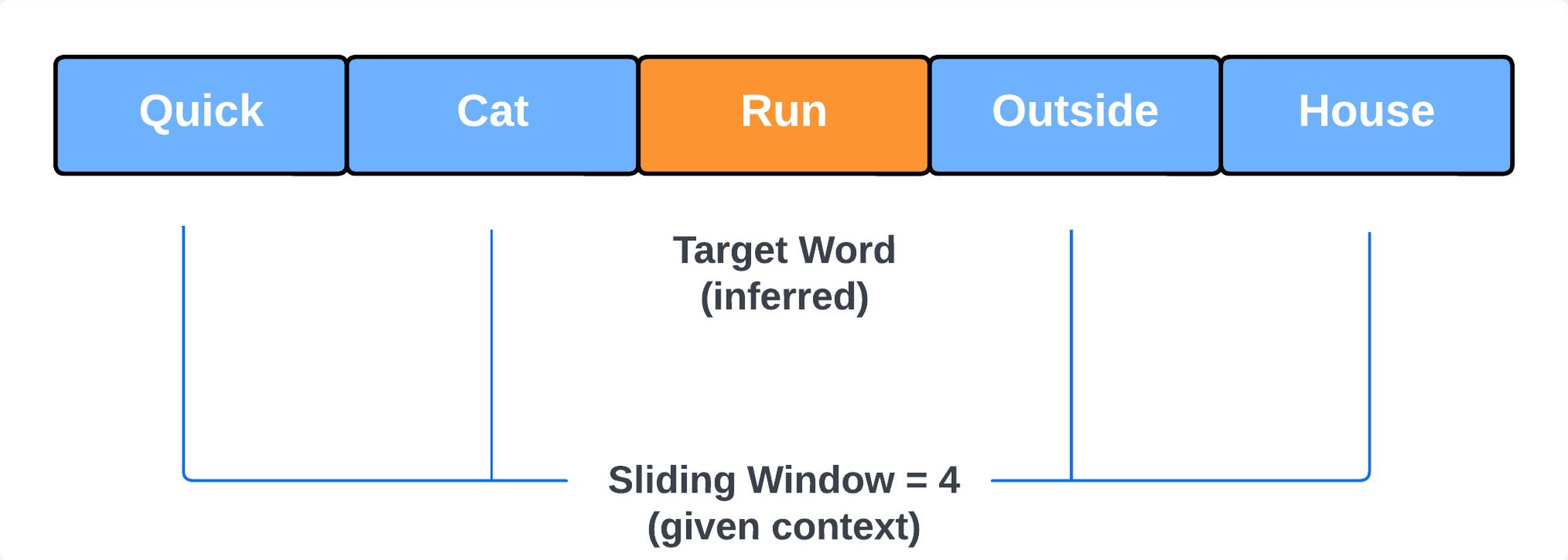
Advantages of CBOW
- Efficiency: CBOW tends to train faster than other models.
- Low Memory Footprint: It uses memory efficiently, which is beneficial for large datasets.
- Performance with Frequent Words: CBOW is particularly good at handling words that appear frequently in the dataset.
Skip-Gram
Architecture Overview
Skip-Gram is another feed-forward neural network model under the Word2Vec family. It is an inverse of CBOW - it predicts context words from a given target word.
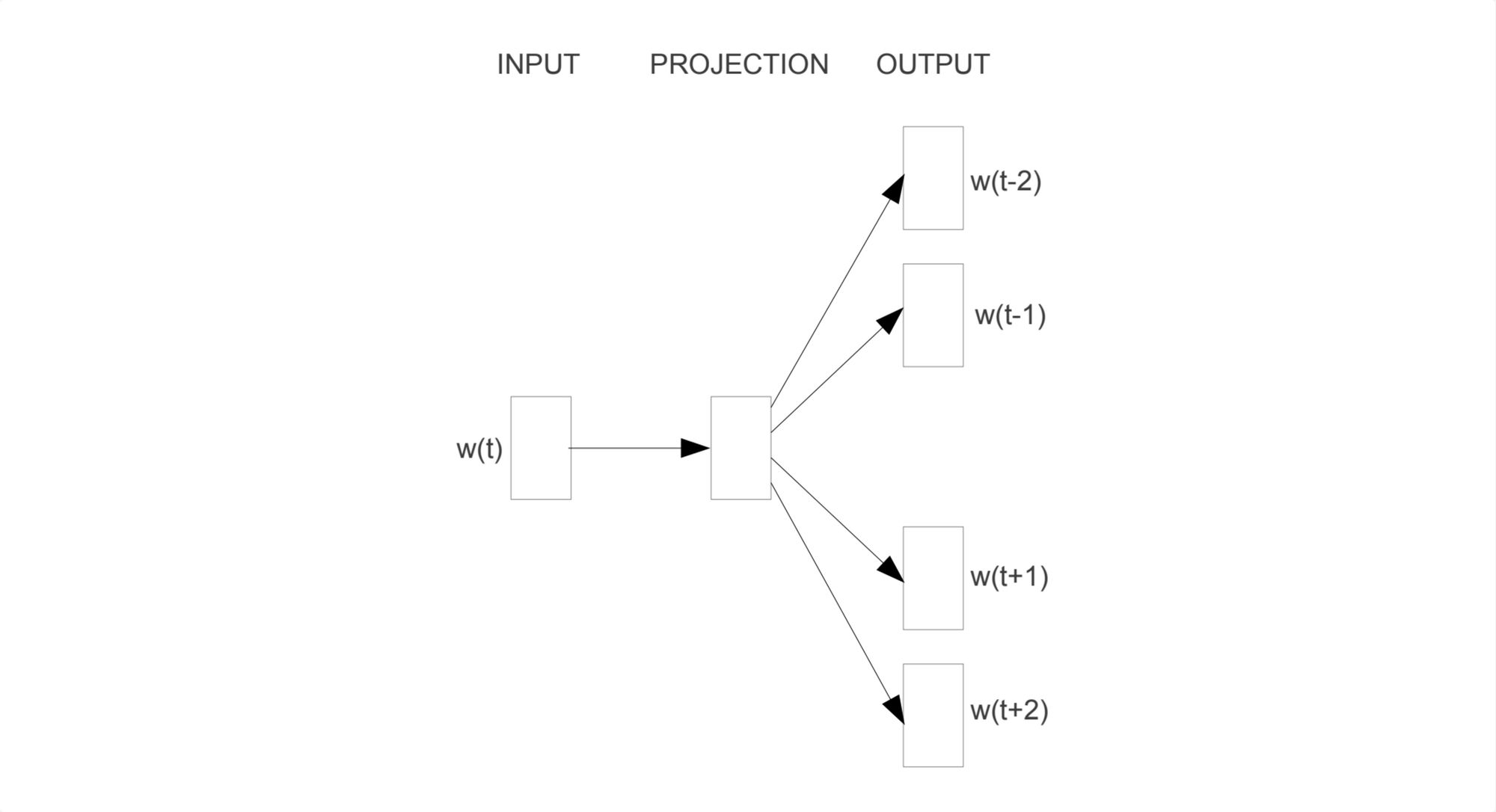
Input Layer
- Target Word: This is the word for which we want to predict the surrounding context. It's encoded as a one-hot vector.
The input size is determined in the same way as in CBOW. The only difference is that the sliding window size doesn't affect input size, because we always have only 1 one-hot encoded vector at the input, by design.
Hidden Layer
Unlike CBOW, where multiple context words are aggregated into a single representation, Skip-Gram starts with just one word. Therefore, there's no need for summing or averaging in the hidden layer. The transformation from the input layer to the hidden layer is a direct retrieval of the word embedding for the input word.
Training Objective
The primary goal during training is to adjust the model's weights to maximize the probability of predicting the correct context words for a given target word.
Example
Consider the sentence: "A quick cat is running outside the house." If "Run" is our target word, Skip-Gram might predict context words like "Quick," "Cat," "Outside," and "House," depending on the window size.
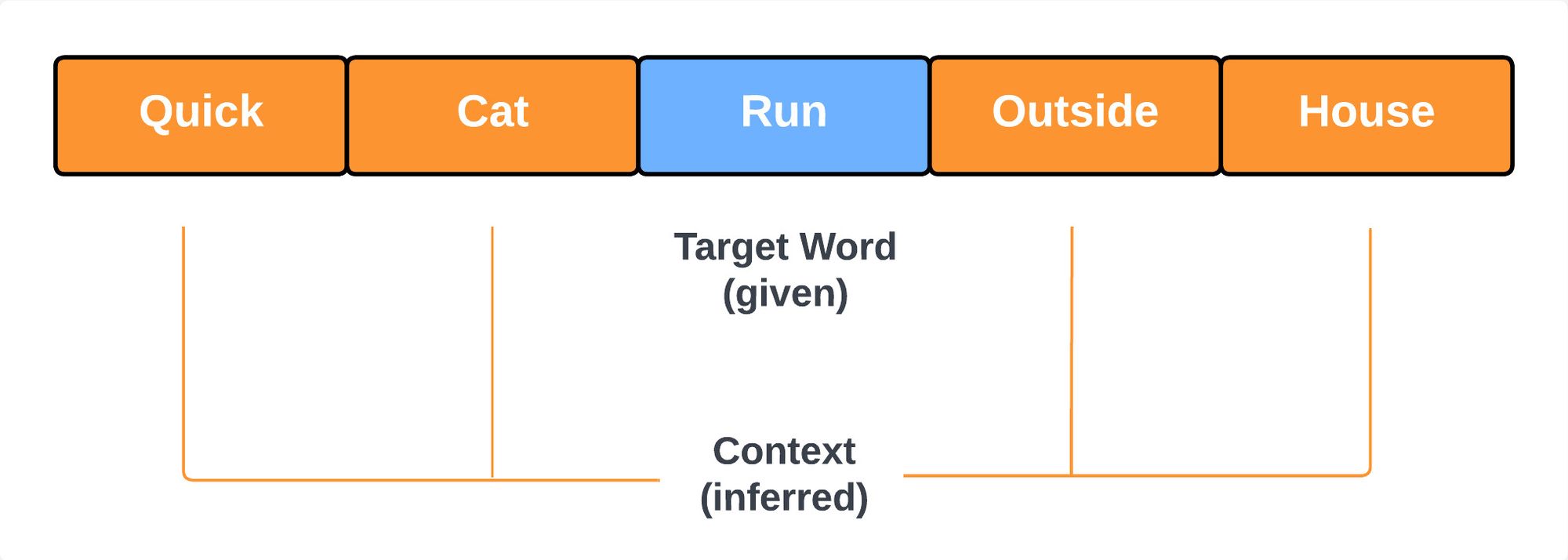
Advantages of Skip-Gram
- Performance with Rare Words: Skip-Gram often provides better representations for less frequent words or phrases compared to CBOW.
- Larger Contexts: It tends to handle larger contexts (bigger window sizes) better than CBOW because it predicts multiple context words from a single target word.
Vector Operations
-
Arithmetics:
-
Use Case: Semantic Discovery & Conceptual Inferences
-
Example 1: Product Recommendations: If an e-commerce platform knows that a user has bought "bread" and "peanut butter", using vector arithmetic, it can infer that the user might also be interested in "jelly" (because "bread" + "peanut butter" - "sandwich" might be close to "jelly" in the vector space).
-
Example 2: Content Creation & Assistance: For writers or content creators, vector arithmetics can help in brainstorming. If they're writing about "winter" and "beach", the result of the vector arithmetic might suggest concepts like "tropical vacations" or "winter sun destinations".
-
Example 3: Cultural Analysis: By analyzing how certain concepts relate to each other across different languages or cultures, researchers can gain insights into cultural biases or perspectives. For instance, understanding how "man" relates to "work" versus how "woman" relates to "work" in different languages can provide insights into gender roles in different cultures.
-
-
Cosine Similarity: Measure the semantic similarity between two word vectors.
- Use Case: Document Retrieval & Recommendation Systems
- Example: In search engines, when a user inputs a query, the search engine can use cosine similarity to find documents that are semantically similar to the query, ensuring that the most relevant documents are retrieved. Similarly, in recommendation systems, if a user likes a particular article or product, cosine similarity can help find other articles or products that are "similar" and recommend them to the user.
-
Word Analogies: Solve relationships like "man" is to "woman" as "king" is to "____?".
- Use Case: Linguistic Research & Cognitive Analysis
- Example: Linguists can use word analogies to study semantic relationships between words and understand how different languages capture similar relationships. It can also be used in cognitive tests to measure linguistic intelligence.
-
Find Synonyms: Identify semantically similar words based on proximity in vector space.
- Use Case: Text Augmentation & Query Expansion
- Example: In chatbots, if a user inputs a query with a word that the bot doesn't recognize, finding synonyms can help the bot understand the user's intent. Similarly, in search engines, query expansion can be used to add synonyms to a user's query to retrieve more relevant results.
-
Out-of-Vocabulary Words: Generate embeddings for words not in the original vocabulary by averaging subword embeddings.
- Use Case: Handling Rare Words & Typos in Text Processing
- Example: In sentiment analysis, if a user review contains a rare word or a typo that wasn't in the training data, generating embeddings for such out-of-vocabulary words ensures that the sentiment model doesn't ignore these words and can still make accurate predictions.
-
Sentence/Document Embeddings: Represent entire sentences or documents by averaging word embeddings.
- Use Case: Document Classification & Sentiment Analysis
- Example: In sentiment analysis, entire reviews (sentences or paragraphs) can be converted into vectors using document embeddings. These vectors can then be fed into a classifier to determine if the review is positive, negative, or neutral.
-
Semantic Clustering: Group semantically related words based on their embeddings.
- Use Case: Topic Modeling & Content Categorization
- Example: News agencies can use semantic clustering to group news articles into different categories or topics based on their content. This helps in automatically categorizing articles into sections like "Sports", "Politics", "Entertainment", etc.
Implementation in Python
Dive into a hands-on demonstration of Word2Vec vector operations using the Gensim library in my Python notebook. Explore the code and examples in detail:
Word2Vec with Gensim: Python Notebook
Visualization
Tensorflow has created an interactive visualization for Word2Vec. I recommend you try it to get an idea how word embeddings are places in 3D/2D space.
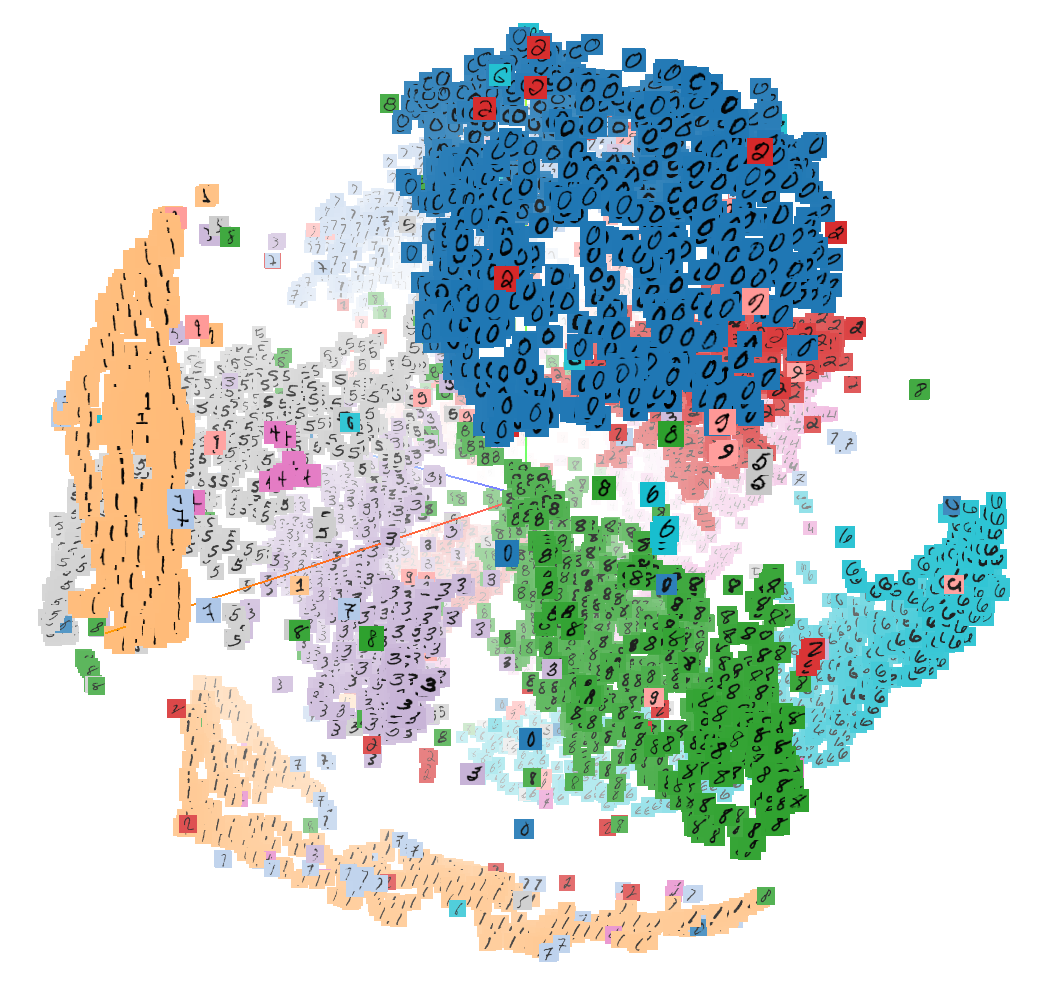
Conclusion
Word2Vec has revolutionized the way we represent and understand words in machine learning. By converting text into dense vectors, it captures intricate semantic relationships and offers advantages over traditional methods. As you delve deeper into natural language processing, harnessing the power of Word2Vec can be a game-changer for your projects.